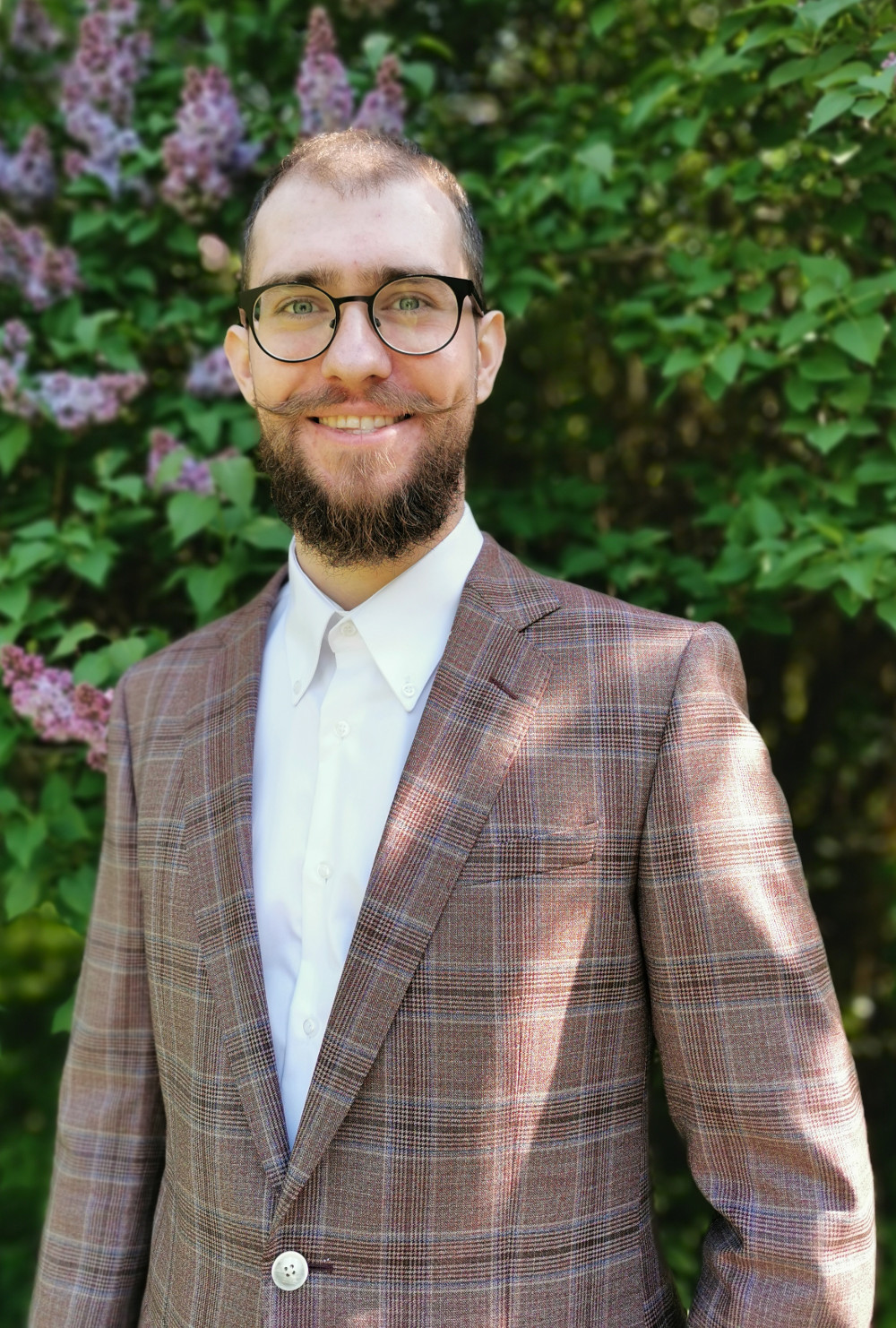
Anton Pershin
Researcher / Team Lead
I am a researcher at the Saint Petersburg Software Technology Innovation Lab at Huawei. Prior to that, I was working on low-precision computing in the Predictability of Weather and Climate group at the University of Oxford and the long-standing problem of transition to turbulence as a Ph.D. student at the School of Mathematics at the University of Leeds supervised by Cedric Beaume and Steven Tobias.
Email: anton.pershin (at) huawei.com
Huawei Technologies Co., Ltd.Saint Petersburg Research Center
Marata street 69/71, Building A
Saint Petersburg, 191119, Russia
Promotion: Team Lead at Huawei
From the 23rd of June, I am holding a team lead position in the Saint Petersburg Software Technology Innovation Lab at Huawei. Show more
I was promoted to a team lead position for the Mathematical Modelling and Optimization team. As a team, we are going to focus on two big directions: time series analysis and large-scale optimization. While working with telecom time series, we recognized the importance of spatiotemporal dynamics on networks. At the same time, we found out that real network time series are heavily affected by external conditions which may lead to the lack of predictability and cause the low performance of any non-adaptive forecasting model. These problems make up exciting topics for our further research so we are going to gain more knowledge about them making use of both simulators and real-world data. On the optimization side, our team is going to focus on applying distributed optimization to large-scale network optimization problems.
"Training a neural network to predict dynamics it has never seen" now out in Phys.Rev.E
In this paper, written together with Cedric Beaume, Kuan Li and Steven Tobias, we demonstrated that Echo State Network can learn beyond the information provided in the training dataset. Show more
Previous studies have shown how echo state networks (ESNs), a type of recurrent neural networks, can successfully predict both short-term and long-term dynamics of even chaotic systems. This study shows that, remarkably, ESNs can successfully predict dynamical behavior that is qualitatively different from any behavior contained in their training set. Evidence is provided for a fluid dynamics problem where the flow can transition between laminar (ordered) and turbulent (seemingly disordered) regimes. Despite being trained on the turbulent regime only, ESNs are found to predict the existence of laminar behavior. Moreover, the statistics of turbulent-to-laminar and laminar-to-turbulent transitions are also predicted successfully. The utility of ESNs in acting as early-warning generators for transition is discussed. These results are expected to be widely applicable to data-driven modeling of temporal behavior in a range of physical, climate, biological, ecological, and finance models characterized by the presence of tipping points and sudden transitions between several competing states.
"Optimizing the control of transition to turbulence using a Bayesian method" now out in JFM
In this paper, written together with Cedric Beaume, Tom Eaves and Steven Tobias, we studied the nonlinear robustness of a shear flow subject to control. Show more
The nonlinear robustness of laminar plane Couette flow is considered under the action of in-phase spanwise wall oscillations by computing properties of the edge of chaos, i.e. the boundary of its basins of attraction. Three measures are used to quantify the chosen control strategy on laminar-to-turbulent transition: the kinetic energy of edge states (local attractors on the edge of chaos), the form of the minimal seed (least energetic perturbation on the edge of chaos), and the laminarization probability (the probability that a random perturbation from the laminar flow of given kinetic energy will laminarize). A novel Bayesian approach is introduced to enable the accurate computation of the laminarization probability at a fraction of the cost of previous methods. While the edge state and the minimal seed provide useful information about the dynamics of transition to turbulence, neither measure is particularly useful to judge the effectiveness of the control strategy since they are not representative of the global geometry of the edge. In contrast, the laminarization probability provides global information about the edge and can be used to evaluate the control effectiveness by computing a laminarization score (the expected laminarization probability) and the associated expected dissipation rate of the controlled flow. These two quantities allow for the determination of optimal control parameter values subject to desired constraints. The results discussed in the paper are expected to be applied to a wide range of transitional flows and control strategies aimed at suppressing or triggering transition to turbulence.
New position: Researcher at Huawei
From the 7th of October, I am taking a permanent researcher position in the Saint Petersburg Software Technology Innovation Lab at Huawei. Show more
I joined the Mathematical Modelling and Optimization team where I am going to focus on time series analysis applied to telecom data. The nature of telecom time series can be extremely diverse: from radio wave propagation to traffic fluctuations due to people wanderings, from optical power in cables to RAM access. It is curious to get to know how to cope with this diversity and find appropriate tools for them
New position: Postdoctoral Research Assistant at the University of Oxford
From the 1st of October, I am taking a postdoctoral position in the Predictability of Weather and Climate group at the University of Oxford. Show more
Being a part of the project ITHACA «An Information Theoretic Approach to Improving the Reliability of Weather and Climate Simulations» led by Prof. Tim Palmer, I will be working on improving the computational efficiency of weather and climate models using the ideas of mixed-precision arithmetic and information theory. One of the key ideas underlying the project is to adapt weather and climate models in such a way that a multiprocessor system running a model transports and makes computations with only those bits of model variables which bear non-negligible information. This suggests transition from conventional 64-bit floating-point numbers to 32, 16 or even 8 bits which may potentially result in saving a great deal of computational time. Hopefully, this work will help develop very high-resolution climate models with a 1km grid whose runs on the forthcoming exascale computers will only be feasible if their computational cost is drastically reduced.
PhD thesis defended
The thesis, written under the supervision of Cedric Beaume and Steven Tobias, is devoted to the assessment and control of transition to turbulence in plane Couette flow. Show more
This work can be divided into two parts: transition to turbulence from the spatially localised states and assessment of the laminar flow robustness using the laminarisation probability. In the first part, we thoroughly describe the dynamics of exact localised solutions (equilibria and travelling waves) in a simple channel flow (plane Couette flow) and then study how their dynamics changes when we start oscillating the walls of the channel. In the second part, we develop a general approach to the characterisation of the nonlinear robustness of linearly stable laminar flows based on the calculation of the laminarisation probability, i.e., the probability that a random perturbation decays as a function of its kinetic energy. We show that the laminarisation probability can successfully be used to find optimal control configurations aimed at eliminating or provoking turbulence.
"A probabilistic protocol for the assessment of transition and control" now out in JFM
In this paper, written together with Cedric Beaume and Steven Tobias, we suggested a new way to assess the nonlinear robustness of laminar flows using the laminarisation probability. Show more
The laminarisation probability denotes the probability that a random perturbation (RP) decays as a function of its kinetic energy. By generating a large number of RPs for multiple energy levels and counting decaying (laminarising) and transitioning ones, we approximated the dependence of the laminarisation probability on the kinetic energy of RPs in a simple shear flow, plane Couette flow, at supercritical Reynolds numbers. This allowed us to study how the nonlinear robustness of the laminar flow to perturbations is affected when we change the Reynolds number or impose a control action. We believe that the suggested protocol is particularly useful for the control design: one could use the laminarisation probability as a measure of the robustness of the laminar flow and, thus, seek to maximise/minimise it by tuning the control parameters. The key issue here is associated with the computational restrictions which we plan to address in future works by making use of the Bayesian inference. Despite the fact that the protocol is presented in the context of one of the canonical shear flows, it can easily be adapted for other nonlinear systems featuring finite-amplitude instability.
"Dynamics of spatially localized states in transitional plane Couette flow" now out in JFM
This paper, authored by Cedric Beaume, Steven Tobias and myself, concerns the dynamics of exact localised solutions in a spanwise-extended domain. Show more
Transition to turbulence is known to occur via spreading of turbulent spots -- small localised patches of turbulence embedded in the laminar flow. To study the phenomenon of transition, one needs to find a way to generate them. For instance, turbulent spots could be produced from the random noise, turbulent states or modeled analytically. In this paper, we suggest exact localised states as an alternative set of initial conditions and investigate their dynamics at pre-transitional Reynolds number. Our localised initial conditions are (numerically) exact solutions of Navier–Stokes equation living on the branches of homoclinic snaking, a beautiful bifurcation pattern well-studied in the Swift–Hohenberg equation and doubly diffusive convection. We present a schematic of parameter space which summarizes the various regimes localized initial conditions exhibit involving oscillatory dynamics, spot splitting and front growth.